The Importance of Semantic Segmentation Labeling Tools in Data Annotation
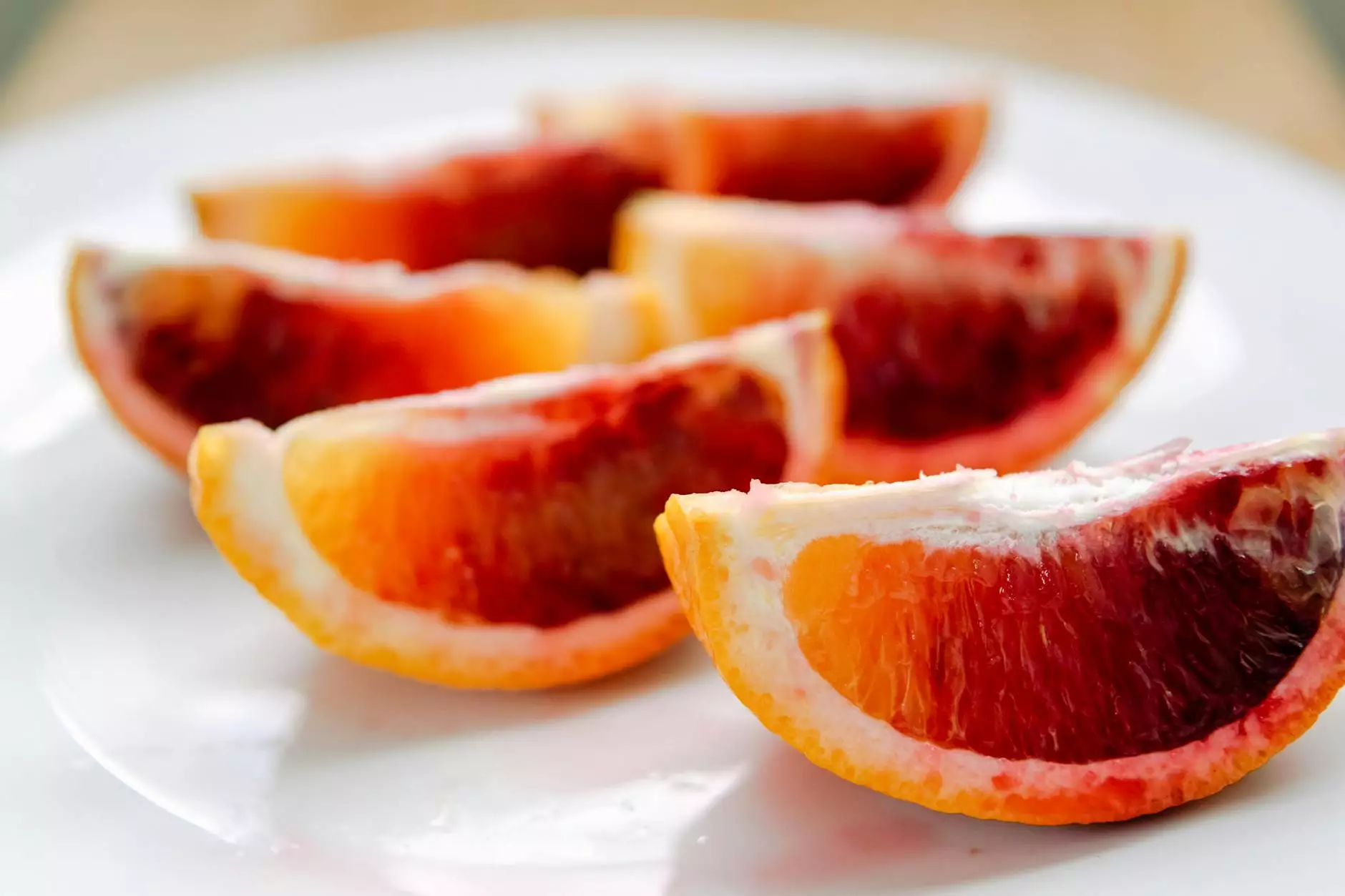
In today's data-driven world, the importance of accurate data labeling cannot be overstated. A crucial aspect of data labeling is semantic segmentation, which involves classifying each pixel in an image to facilitate advanced machine learning models. The semantic segmentation labeling tool has emerged as a vital part of data annotation, offering unprecedented accuracy and efficiency across various sectors. This article will delve deep into the intricacies of semantic segmentation, its applications, and why tools like those provided by Keylabs.ai are essential for businesses aiming to leverage data effectively.
Understanding Semantic Segmentation
Semantic segmentation is a computer vision task that assigns class labels to every pixel in an image. Unlike traditional image classification, which labels the entire image, semantic segmentation enables a more detailed understanding of the content within an image. This level of granularity is vital for applications in various fields, including healthcare, autonomous vehicles, and environmental monitoring.
How Semantic Segmentation Works
At its core, semantic segmentation splits an image into semantically meaningful segments. Each segment is classified with a label, helping machines understand the context of the image. Here's a step-by-step overview of how the process works:
- Data Collection: Gather a diverse and representative set of images that cover all classes of interest.
- Data Annotation: Utilize a semantic segmentation labeling tool to annotate the images by marking the pixels belonging to different classes.
- Model Training: Use the annotated dataset to train a deep learning model capable of performing segmentation tasks.
- Model Evaluation: Assess the model’s performance on a validation dataset to ensure it can accurately predict segmentations on unseen data.
The Role of Semantic Segmentation Labeling Tools
While the concept of semantic segmentation may seem straightforward, the actual process requires meticulous effort and precision. This is where semantic segmentation labeling tools come into play, and they offer several distinct advantages:
1. Enhanced Accuracy
Tools like those available on Keylabs.ai are designed to minimize human error during the annotation process. They provide a user-friendly interface, enabling annotators to focus on the details without getting lost in complexity. Increased accuracy in labels directly influences the model's predictions, leading to better outcomes.
2. Improved Efficiency
Manual data annotation is often time-consuming, but with specialized semantic segmentation labeling tools, the speed of annotation increases significantly. Features such as batch processing and automated suggestions allow annotators to complete their tasks much faster, which can lead to significantly reduced project timelines.
3. Scalability
As the volume of data increases, it becomes imperative for businesses to have scalable solutions. Semantic segmentation tools accommodate large datasets and allow for streamlined workflows that adapt as projects grow. This is particularly beneficial for companies seeking to expand their datasets without a proportional increase in labor costs.
Industry Applications of Semantic Segmentation
The applications of semantic segmentation and its labeling tools are vast, serving as a backbone for several industries:
1. Autonomous Vehicles
In the realm of automated driving technologies, semantic segmentation plays a critical role in the identification of objects on the road. From pedestrians to road signs, accurate segmentation allows self-driving cars to navigate safely and efficiently.
2. Healthcare
Medical imaging relies heavily on semantic segmentation for diagnostic purposes. By accurately segmenting areas of interest in MRI or CT scans, medical professionals can better identify tumors, lesions, and other abnormalities, ultimately leading to improved patient outcomes.
3. Agricultural Technology
Precision agriculture utilizes semantic segmentation to analyze crop health. By examining images of fields, farmers can identify areas needing attention, effectively allocating resources such as water and fertilizers to optimize yield.
4. Robotics
In robotics, semantic segmentation aids in object recognition and scene understanding. Robots equipped with semantic segmentation capabilities can perform tasks like sorting, fetching, and navigating complex environments.
Choosing the Right Semantic Segmentation Labeling Tool
When considering a semantic segmentation labeling tool, businesses need to evaluate various factors to ensure they select the best fit for their needs:
1. User Experience
The interface of the tool should be intuitive and easy to navigate. A good user experience reduces the training time for new annotators and enhances productivity.
2. Automation Features
Look for tools that offer automation options, such as pre-existing labels or intelligent suggestions based on previously annotated images. Such features can significantly cut down annotation time.
3. Integration Capabilities
The ability to integrate with existing data management systems and machine learning frameworks is essential. Tools that support easy data export and model training streamline the workflow.
4. Support and Documentation
Comprehensive support and documentation are crucial, especially for organizations new to data annotation. Ensure that the tool you choose has a solid support system in place.
Future Trends in Semantic Segmentation
The field of semantic segmentation is ever-evolving. Here are some anticipated trends that may shape the future of this technology:
1. Advanced AI Integration
As artificial intelligence continues to advance, we can expect to see more sophisticated AI-driven tools that not only assist in segmentation but also learn and adapt from user inputs over time.
2. Real-time Segmentation
Real-time capabilities in semantic segmentation will become increasingly important, particularly in applications such as augmented reality and autonomous systems where timely data processing is crucial.
3. Enhanced Collaboration Tools
Future semantic segmentation tools will likely focus on collaborative features, enabling multiple users to annotate data simultaneously and share insights, ultimately speeding up the annotation process.
The Bottom Line
The use of semantic segmentation labeling tools is set to revolutionize the way businesses approach data annotation. As sectors like autonomous vehicles, healthcare, agriculture, and robotics increasingly rely on accurate pixel-level understanding of images, the need for efficient and reliable tools is greater than ever. By adopting advanced solutions like those available through Keylabs.ai, companies can ensure they harness the full potential of their data.
Investing in semantic segmentation not only enhances the quality of data but also drives innovation, efficiency, and competitive advantage in an ever-growing digital landscape. Embrace the power of semantic segmentation labeling tools and transform your data annotation approach today!