The Ultimate Guide to Labeling Tool Machine Learning
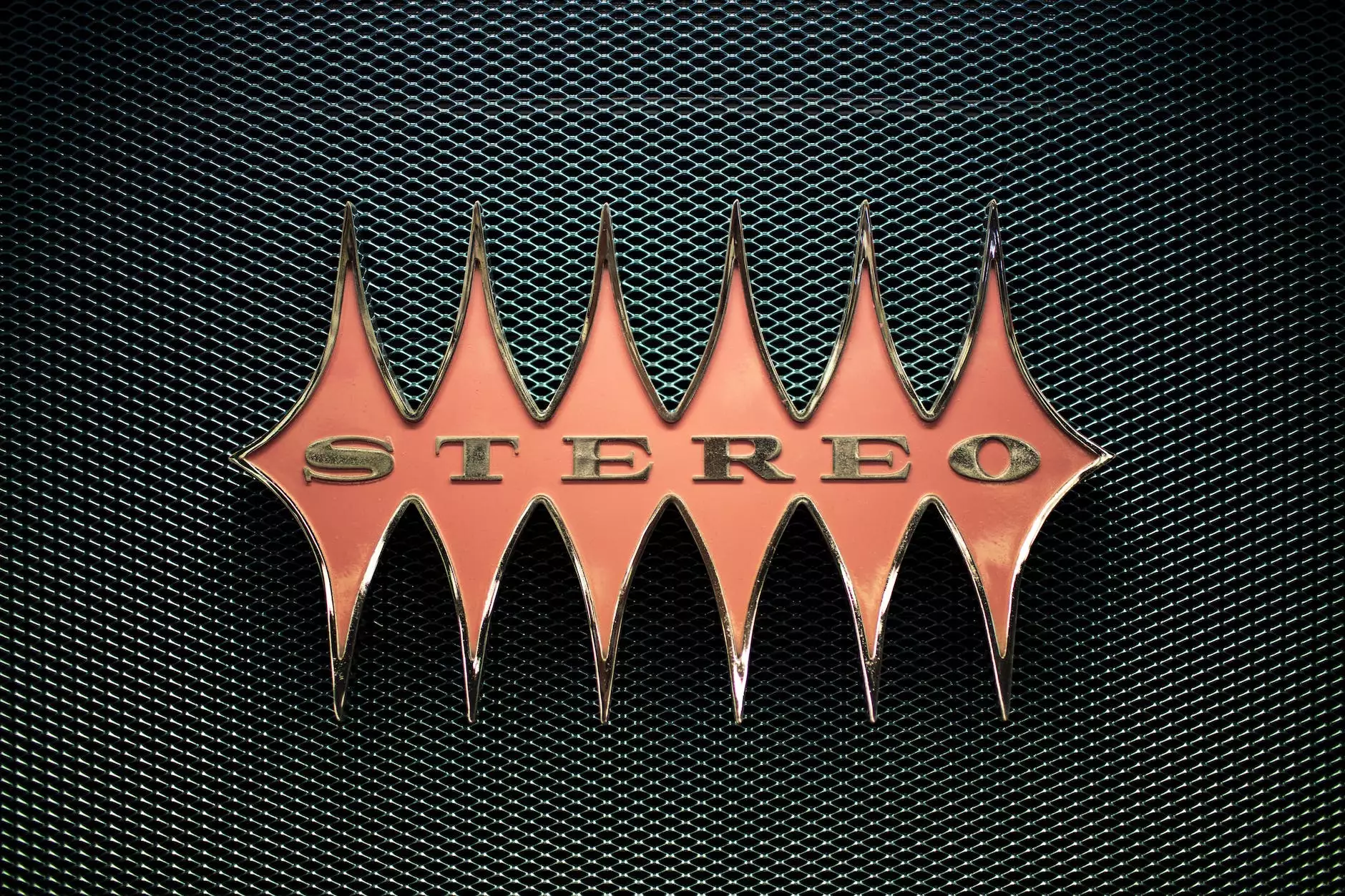
In the age of data science and artificial intelligence, the importance of high-quality annotated data cannot be overstated. Machine learning relies heavily on training data that is accurately labeled, making robust labeling tool machine learning solutions indispensable for various industries. This article will explore the significance of labeling tools in the machine learning landscape, discussing their features, benefits, and future trends.
What Are Labeling Tools in Machine Learning?
Labeling tools are software applications designed to assist in the data annotation process. These tools enable users to assign meaningful labels to raw data, which can range from images and videos to text and audio. In machine learning, the accuracy of the output model is directly dependent on the quality of the input data, emphasizing the need for reliable data annotation tools.
Key Features of Labeling Tools
When selecting a labeling tool, several key features should be evaluated:
- Automated Labeling: Many modern tools utilize machine learning algorithms to automate the labeling process, significantly speeding up the workflow.
- User-Friendly Interface: A simple and intuitive interface is crucial for improving team collaboration and reducing training time.
- Integration Capabilities: The ability to integrate with other machine learning frameworks and data management systems is essential for seamless operations.
- Collaboration Tools: Features that support real-time collaboration and feedback between team members can enhance productivity.
- Data Security: Since annotated data might be sensitive, robust security measures are necessary to protect it.
- Quality Control Mechanisms: These tools should offer built-in quality checks to ensure labeled data adheres to defined standards.
The Benefits of Using Labeling Tools in Machine Learning
Implementing an effective labeling tool machine learning strategy brings numerous benefits:
1. Enhanced Data Quality
The accuracy of data annotations directly affects model performance. With the right tools, organizations can achieve a higher level of precision in labeling, thereby improving the quality of their data. This ultimately leads to more reliable machine learning models.
2. Increased Efficiency
Labeling tools, particularly those equipped with automation capabilities, significantly reduce the time required for data annotation. This means faster turnaround times for projects, enabling companies to deploy machine learning models more swiftly.
3. Scalability
As data volumes grow, the ability to efficiently scale data annotation efforts becomes critical. Labeling tools enable teams to handle larger datasets without compromising quality, ensuring that enterprises can keep pace with their data needs.
4. Cost-Effective Solutions
Outsourcing data annotation can be costly. By utilizing in-house labeling tools, organizations can save money while fostering a deeper understanding of their data.
Industry Applications of Labeling Tools
The versatility of labeling tools means they find applications across various industries:
1. Healthcare
In healthcare, labeling tools are essential for annotating medical images, patient data, and clinical reports. Accurate labeling aids in developing machine learning models that can assist in diagnostics and treatment recommendations.
2. Automotive
Self-driving cars rely heavily on labeled data for training their perception systems. Labeling tools are used to annotate images and sensor data, ensuring algorithms correctly interpret surroundings.
3. Retail
In retail, labeling tools help in recognizing products in images, analyzing customer feedback, and predicting market trends through sentiment analysis of textual data.
4. Finance
Financial institutions utilize labeling tools for risk assessment, fraud detection, and customer behavior analysis. Accurate labeling of transactions and customers leads to better predictive models.
Best Practices for Using Labeling Tools
To maximize the effectiveness of labeling tools, organizations should adopt best practices:
1. Define Clear Labeling Guidelines
Establishing clear and comprehensive labeling guidelines is crucial. These guidelines help maintain consistency and ensure that team members adhere to the same standards when labeling data.
2. Train Your Team
Proper training on how to use labeling tools and the importance of accurate labeling is essential for achieving high-quality outputs. Regular workshops can help ensure that all team members are up-to-date on best practices.
3. Utilize Quality Control Processes
Incorporating regular quality control checks can help identify any discrepancies in labeling, allowing teams to address issues promptly and maintain data integrity.
Future Trends in Labeling Tools
The landscape of data annotation is constantly evolving, and several trends are shaping the future of labeling tools:
1. AI-Powered Labeling
As artificial intelligence continues to advance, labeling tools will increasingly harness AI algorithms to automate and enhance the labeling process. This will lead to quicker, more accurate annotations.
2. Improved Collaboration Features
Future labeling tools will likely focus on enhancing collaboration features to facilitate better communication among data scientists, domain experts, and annotators.
3. Customizable Solutions
Organizations will seek more customizable labeling solutions that can be tailored to meet specific industry requirements, ensuring that they get the most out of their data annotation efforts.
Conclusion
In summary, labeling tool machine learning is a vital component of the data-driven world we live in today. With the right tools, organizations can enhance data quality, improve operational efficiency, and remain competitive in their respective fields. As machine learning continues to evolve, the demand for sophisticated labeling solutions will only grow. By staying informed about trends and best practices in data annotation, businesses can harness the full potential of their data and drive innovation forward.
For more information and to explore advanced Data Annotation Tool and Data Annotation Platform solutions, visit keylabs.ai.